Knowledge Graphs- Arrange Data and Leverage Information To Decipher Life Solutions
Shikha SaxenaAug 7, 2020 · 6 min read
With the availability of internet for public use since August 6, 1991, loads of digital information is generated and has multiplied manifolds. Generation and flow through web have created sea of information, about real world entities, on world wide web and it is increasing at a rapid speed, every moment. Solutions to questions have become easy on the net and is readily available, through the mobile and other digital devices. Have you ever wondered about the availability of information whenever you need and answers to all questions are independent of your geographic location? What is behind interlinking of this information? This information is accumulated overtime from billions of websites and pages created by users worldwide. This vast information is overwhelming and leads to confusion. We often doubt about the ambiguity of this information as well. Creation of Knowledge Graph (KG) is an approach to interlink worldwide information and leverage it through research, analysis and programmatic construct to decipher life solutions of multiple domains as in Medicine, Technology, Artificial Intelligence (AI), Social, Forensic Science.
What exactly is knowledge?
Knowledge is all information about real world objects. It may be people, non-living things, businesses, countries, animals, plants and other entities. This comprises of all data attributes as name, address, phone number, locations, physical features, related to an entity and the relationships between this data with other entities to reach specific outputs.
There are three kinds of knowledge about any entity or object:
Physical knowledge
Social knowledge
Logic o-mathematical knowledge.
Two major classification of knowledge are
Tacit knowledge: It is experience based, abstract and is non codified
Explicit knowledge: It can be codified in documents through computer language codes.
Concept of knowledge and its graph can be understood by example of growth and development of a brain from the birth onwards. How the innocent, blank, knowledge less brain of a baby, gains experiences and hence knowledge gradually through visuals, voice, olfactory and sound perceptions, and other sources and connects all information and data points to start recognizing parents, family members and daily objects.
Nascent brain starts building up knowledge graph by classifying knowledge gathered, through different sources, and starts naming and storing all information in designated areas of memory, for future use. It keeps building vocabulary on this gathered information, try to connect, compare and create a pattern.
Brain, later leverages gained information, to apply in life functions and find solutions for routine activities. This is the process of learning! And eventually gaining experience and intelligence to become self-sufficient to decipher knowledge and utilize it to face future problems!
This concept of taxonomy, ontology and knowledge base creation is also being used in AI to find intelligent solutions.
What is the meaning of Ontology?
Actually, Ontology is a philosophical approach in metaphysics that deals with the nature of existence or being. If a thing exists it can be classified in different categories based on its differences and similarities to other entities, it is compared with.
In this approach knowledge base created codifies some information and then an ontology is developed to reflect connections between different data elements. Ontology incorporates the concept of naming objects (taxonomy) and entities, assigning classes and attributes, collecting information and preparing Knowledge base. It also allows systems to communicate with each other through rich context and interlinked attributes in knowledge base.
Not only this one, it also communicates and works with multiple systems through workflows, processes and other program constructs to fully realise, ingest information and provide valuable insights.
What exactly is Knowledge Graph?
Knowledge Graph acquires and integrates information into a construct, an ontology and apply a reasoning to derive a new knowledge and insight from it. KG is set of data- points linked together by relations amongst a domain, it can be people (as in Facebook platform and other social media platforms), a business (as in Netflix, Amazon) or an entity. Knowledge graphs can be built automatically through users and their information on the world wide web.
Google Knowledge Graphs came into existence on May 16, 2012 and since then, incoming data have created accurate and structured knowledge panels and created connections and platform of people, places, things and entities.
Study of these graphs reveal important information and can result in great search results and insights when a query is activated through search engines as Google, Yahoo, Bing.
Knowledge graphs have wealth of information that Google uses with its cutting-edge algorithms, easy interfaces and great user experiences, to show searchers how entities are interconnected.
Google reveals accurate and precise information in search results. Google Knowledge panels are rich results which show up on the right-hand side corner, on the Google Search Results page. Panel shows up rich, accurate and precise information about the entity you searched for. Also keeps on updating the information for accuracy. Provide answers to user questions, research information, locating business entities, keeping track of inventories, review management and so on.
Knowledge Graphs are considered as data architecture with nodes of preserved information, which are interconnected with respect to some relations or attributes. These data structures can be used or ingested by algorithms or neural nets for machine learning in AI, by performing tasks as classification, clustering and regression.
Knowledge in these graphs is categorized as general knowledge, site specific and domain specific knowledge. General knowledge is site and domain independent and can be acquired and distributed by various sources on the web.
Knowledge Graphs are the results of Google’s continuous efforts to organize worldwide information and put to use by all, through the application of various query algorithms to give useful insights. Its Google’s own database created through world wide web searches, through billions of searches worldwide and systematically arranged, to be available to use. It has relevant and accurate information about people, place, things and businesses.
Usage of planned keywords, search engine optimization tools and techniques and serial updates are some of the efforts to keep knowledge and hence data relevant and structured. It is made available as search result on web-pages once user type strings of words or sentences as a question. Google’s interlinked Knowledge graphs help answer the search within fraction of seconds.
What Algorithms are active behind Knowledge Graphs?
Knowledge Graphs use two types of algorithms:
Constructive algorithms: It stores and rearranges all unstructured data into structured data with graph of concepts and relationship between entities and attributes. This interlinks the data by comparing and finding relations between concepts.
Query algorithms: It answers the questions or searches of the users by ingesting available data and finding relevant results from the attributes of the knowledge graph.
Though Google knowledge graphs try to furnish accurate information about a query sometimes disparities and irrelevance of the data is observed.
Unmatched results and irrelevant information are the biggest challenges for knowledge integration. It is an area to be deep dived into and resolved.
How knowledge graphs are created?
Creating a graph involves text mining by use of sources of natural language as web-pages, Named Entity Recognition (NER) and Natural Language Processing (NLP) techniques as conferencing and part of speech recognition to find correlations.
Finding relations in text data is difficult task, It involves a lot of intelligence, intuition, prediction and manual focus and concentration.
Therefore, techniques of applying predefined schema and pattern recognition rules are used to establish connection in concepts.
Initially Google knowledge Graph used semi structured Wikipedia and structured databases as Metaweb’s freebases to establish interlinking of concepts. Then Google scaled up the 12 million concepts of Metaweb to 540 million concepts with 18 billion linkages and factual connections between them, after acquiring Metaweb.
Text mining and manual data curation is time consuming on a web scale, therefore Google is using a new technique in its next generation knowledge graph- called Knowledge Vault. This technique is based on- Probabilistic Knowledge Fusion.
Tips to create Knowledge Graph
Creation of knowledge Graph involves text mining and additional intuitive cleverness!
This will remove the inevitable disparities and build a graph with accurate, relevant facts of interlinked concepts, entities with true established relationships. Concepts to be considered during KG creation are as follows:
Ontology and versioning mechanism: These are needed to clarify main object, to maintain consistency.
Named Entity Linking: The extraction of named entities and their interlinking to other KGs.
Slot filling: Once entity is identified, start filling slots as address, mail, phone number, city, activity domain.
Data Clean-up: Data de-duplication, spelling corrections, removal of mistakes and data clean-up is important.
Linking: Linking data with other KG will provide additional data about entities.
Publishing: Publishing data as linked data. Some formats are acceptable that include JASON LD.
Some nice interface plus JASON LD for adoption and serving of this data may furnish fruitful results!
WRITTEN BY
Shikha Saxena
A Technical Writer, an artist and blogger by choice. Passionate about reading , writing and editing. http://www.shikhasaxena.com and https://www.dnabox.co/
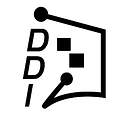
DataDrivenInvestor
empowerment through data, knowledge, and expertise. subscribe to DDIntel at https://ddintel.datadriveninvestor.com
As published in Medium:
Share Your Thoughts